
Don’t Get Left Behind! Take FinOps to the Next Level with ML
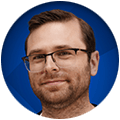
CTO and Co-founder
If you’re having trouble keeping cloud costs down as your business grows, you’re not alone. As cloud environments become more complex, optimizing costs becomes a challenge. Traditional human monitoring struggles to keep up with the influx of data, leading to unexpected cost escalations that negatively impact FinOps performance. This situation further burdens engineers with additional tasks like forecasting usage and adjusting allocations, adding to their already demanding to-do list.
Fortunately, Machine Learning (ML) comes to the rescue by swiftly analyzing vast amounts of data and making intelligent decisions for various critical scenarios. In the realm of FinOps optimization, ML plays a crucial role in the following aspects:
- Recommending cost-effective pricing models and instance types : Leveraging historical data, ML assists organizations in selecting optimal options for greater efficiency, suggesting suitable instance types and pricing models that can help reduce costs.
- Analyzing usage patterns and identifying underutilized resources: ML helps organizations identify inefficiencies and reduce costs by examining usage patterns and pinpointing resources that are not fully utilized.
- Analyzing usage patterns for accurate prediction of future resource requirements: ML analyzes cloud usage to predict future resource requirements accurately. This helps organizations plan ahead and allocate resources optimally, avoiding unexpected spikes in costs.
- Identifying specific aspects that drive costs in the cloud: ML enables organizations to focus their optimization efforts on high-cost areas, providing insights into the specific aspects that contribute most to cloud expenses. This targeted approach maximizes savings potential.
Overall, ML empowers businesses to leverage data-driven insights for efficient cloud cost management, enabling them to rectify inefficiencies, reduce expenses, and make informed decisions for improved financial performance in the cloud.
Adoption of ML to Advance FinOps Initiatives
Because of these benefits, organizations are catching on to the importance of ML in optimizing FinOps.
According to Battery Ventures’ State of Cloud Spending Report, a significant majority of companies (86%) have future plans to adopt ML-based solutions for FinOps and Cloud Cost Optimization within the next five years. This indicates a growing recognition among businesses of the benefits offered by ML in enhancing the return on investment (ROI) of their cloud infrastructure and reducing the workload on engineers.
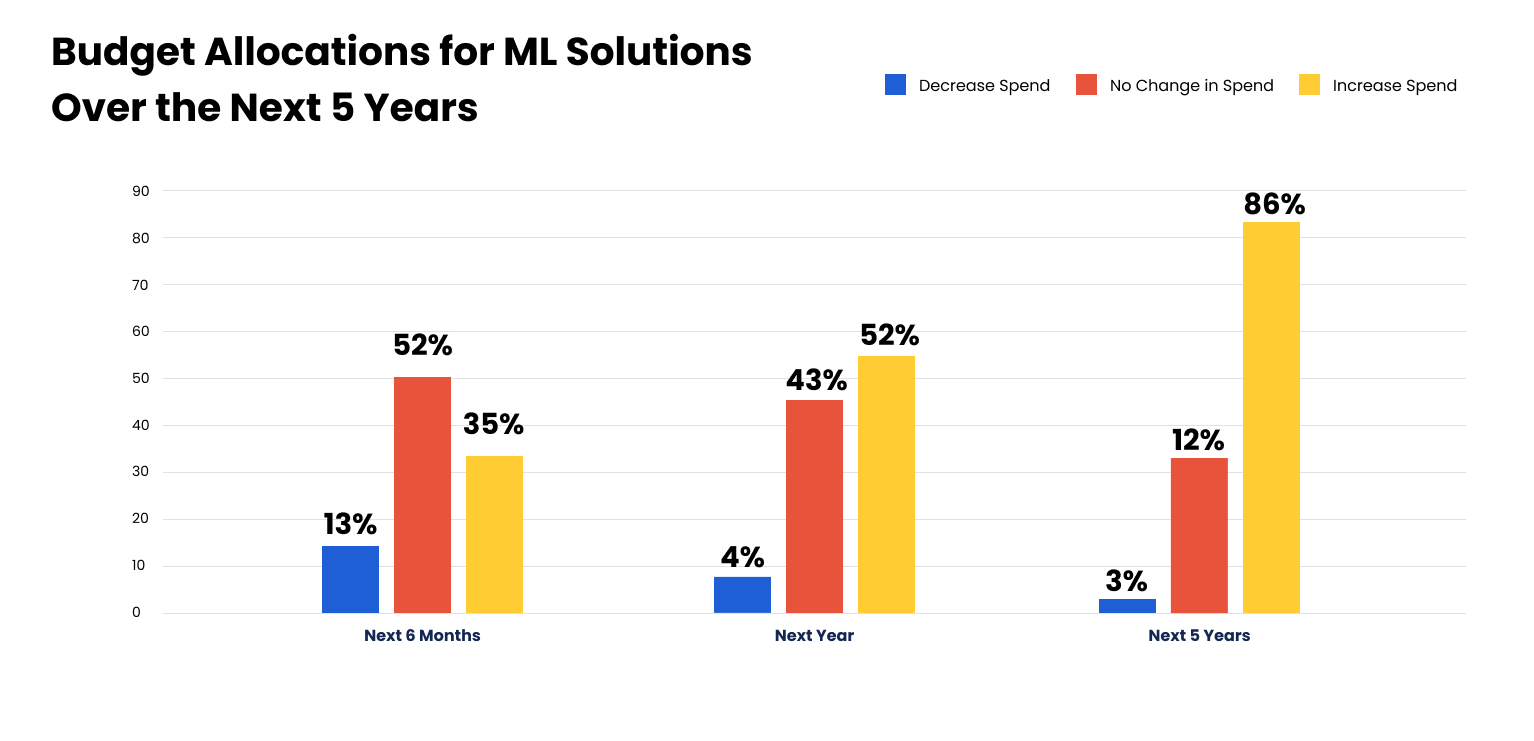
Data originally from Battery Ventures’ State of Cloud Spending Report
Yet, currently, while this technology is prioritized, it has yet to be fully adopted. Research indicates that 95% of CXOs acknowledge the importance of optimizing cloud costs, but only 55% currently utilize ML-based third-party solutions for this purpose. Interestingly, despite the relatively low adoption rate, 31% of CXOs consider ML optimization to be the most critical feature when selecting third-party cloud cost optimization solutions, making it the highest priority feature by the majority of CXOs surveyed.
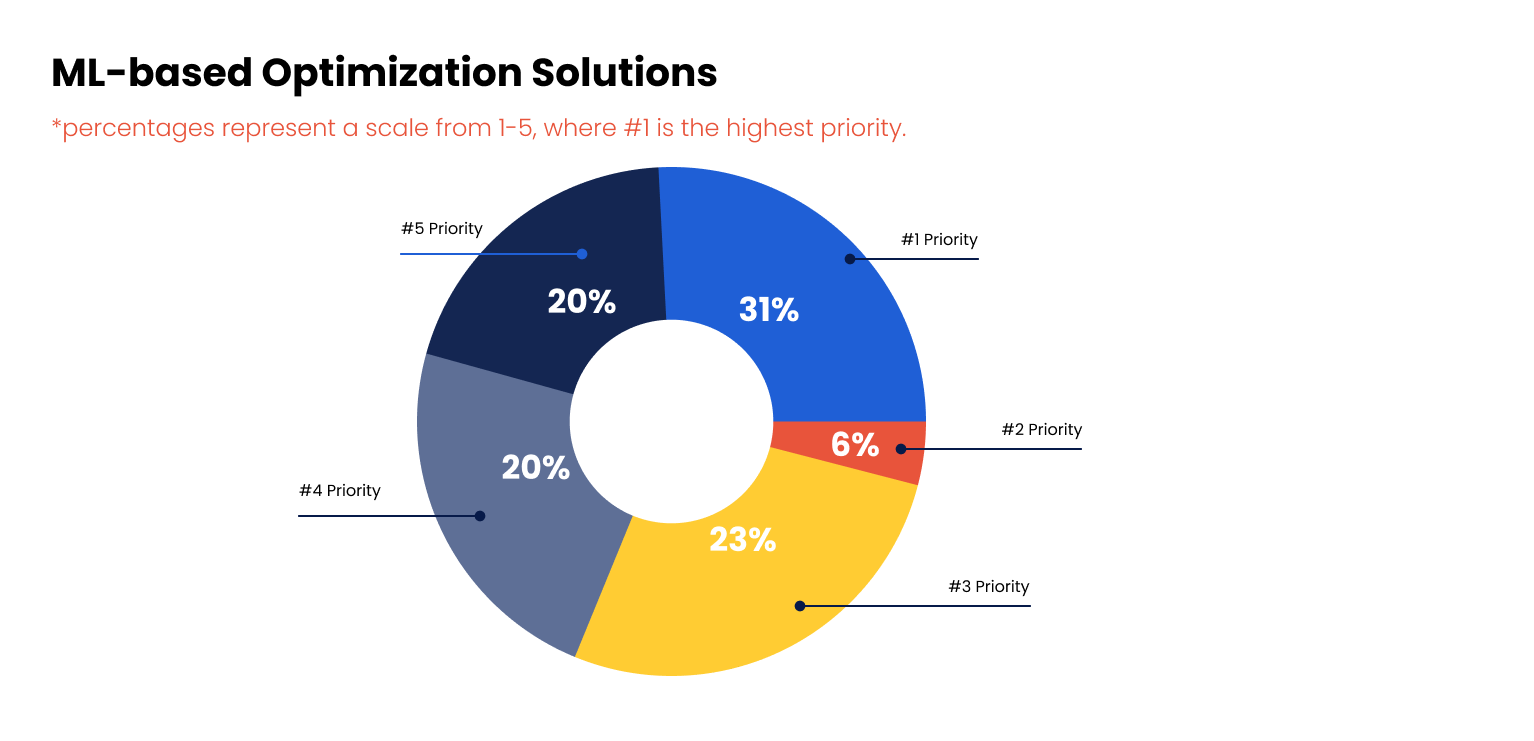
Data originally from Battery Ventures’ State of Cloud Spending Report
This shows that while businesses understand that incorporating ML into FinOps strategies offers a wide range of capabilities to enhance cloud cost efficiency, the technology’s lack of maturity may be delaying its immediate implementation.
Perhaps this can also be attributed to the lack of automation capabilities in the majority of ML solutions. While there are a wide range of tools available in the market, most of them focus on providing visibility and recommendations. These tools excel in delivering real-time insights, pinpointing areas of excessive spending and suggesting ways for organizations to reduce costs. However, human implementation of these recommendations is still required, which means they still take time and effort from humans in order to reduce costs
Transforming Insights into Action
Given the rapid pace of today’s business environment, FinOps practitioners require more than mere recommendations.
In order to fully leverage the advanced potential of machine learning (ML) for FinOps optimization, it is crucial for automation capabilities to be a key component of any ML solution. Automation goes beyond providing recommendations and insights; it enables real-time action to be taken instantly, resulting in cost reduction without requiring any effort or input from engineers.
This technology provides the following benefits:
- Eliminating Human Error: ML enables data-driven decision-making, incorporating real-time insights to deliver precise and reliable cost optimization.
- Minimizing Manual Work: Automation powered by ML relieves the manual workload associated with optimizing cloud costs. Tasks such as usage forecasting, allocation adjustments, and manual scaling of disks are all automated, allowing cloud operations teams to save valuable time and focus on other crucial activities.
- Maximizing Savings: ML algorithms are constantly active, operating around the clock to promptly react to unforeseen changes. This capability allows it to achieve substantial savings compared to solutions that lack ML integration.
Final Thoughts
Traditional human monitoring struggles to handle the complexity and influx of data, resulting in unexpected costs that impact FinOps performance. As a result, engineers are burdened with additional tasks such as usage forecasting and allocation adjustments, which takes time away from their core responsibilities.
Fortunately, ML offers a solution. It excels in recommending cost-effective pricing models and instance types, identifying underutilized resources, accurately predicting future resource requirements, and identifying cost drivers. This enables FinOps teams to optimize cloud costs without investing too much time and effort.
However in order to fully unlock the potential of ML for FinOps optimization, automation capabilities must be prioritized as mere recommendations alone are insufficient in today’s fast-paced business environment. Automation goes beyond recommendations, taking real-time action to instantly reduce costs without requiring manual effort. This helps FinOps teams take their initiatives to the next level, uncovering cloud savings that were otherwise impossible to reach.
Want to see for yourself how Zesty’s ML can take your FinOps initiatives to the next level? Contact our cloud experts today!
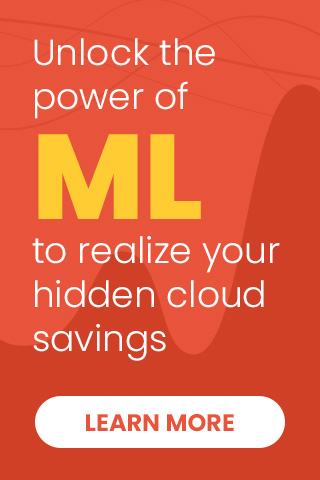
Related Articles
-
How To Maximize Your Reserved Capacity Utilization
May 1, 2024 -
What’s New in AWS? 15 Recent Updates You Need to Know About
April 11, 2024 -
Understanding AWS Compute Waste and How to Prevent It
March 26, 2024 -
Optimize Cost Efficiency for Storage on AWS Batch Workloads
March 19, 2024 -
Amazon EKS Unveils New Pricing for Extended Kubernetes Support
March 11, 2024
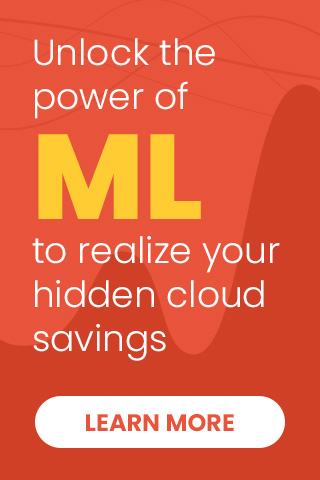